Real-time data isn't real-time if you're still waiting for reports
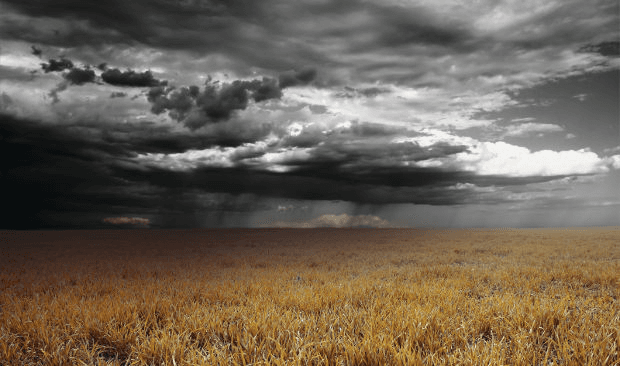
Leo Delmouly
A storm is brewing.
An underwriter refreshes their dashboard. The numbers load, but something feels off. The premium trends look a little strange. Claims are climbing, but is it just a seasonal fluctuation, or is something more systemic happening?
They have questions. But to get answers? That’s another story.
They’ll have to wait.
Even though their company has a state-of-the-art streaming infrastructure, the data they need is still locked behind batch processes, ETL jobs, and reporting tools that force them into predefined, static views. If they want to dig deeper: slice data in a new way, compare different risk models, or explore an emerging trend, they have to request a new report from IT.
By the time they get it, the storm has already passed. The risk is already on the books. And worse? They never even saw it coming.
Kafka is everywhere. But it’s not enough
Event streaming platforms have revolutionized operational data flows in insurance. Claims, policies, transactions, all of it flows seamlessly between microservices, ensuring that critical systems stay in sync.
But when it comes to analytics, Kafka is still vastly underutilized.
Why? Because almost every organization still moves data out of Kafka and into a separate analytics environment before they can generate reports.
Here’s how it typically works:
- Kafka captures events in real-time: a new policy is written, a claim is filed, a payment is processed.
- That data is sent to a storage layer (a data lake or warehouse), where it sits until batch jobs process it.
- Once processed, the data finally makes its way into a BI tool, where underwriters, reinsurers, or finance teams can access it.
This approach breaks the real-time promise of Kafka. The data might be fresh when it enters the pipeline, but by the time it reaches decision-makers, it’s already stale.
What about stream processing? Isn't that real-time?
Many organisations have tried to reduce batch processing latency by using stream processing tools like Flink, KSQL or Kafka Streams. These technologies allow real-time transformations and aggregations, making data available more quickly.
The upside? They're great for reducing the staleness of batch jobs and keeping operational systems up to date.
The downside? They're still rigid and predefined.
Here's why:
- Stream processing requires defining queries and transformations upfront.
- If an underwriter suddenly wants to slice data differently, compare new risk models, or ask an unexpected question, they can't do it dynamically.
- Any change requires modifying stream processing logic, redeploying pipelines or requesting IT to reconfigure queries.
So while stream processing helps with latency, it doesn't enable true ad hoc exploration.
This is why most underwriting and finance teams still rely on batch-processed data lakes and warehouses for deep analysis. But even there, predefined reports force them into static views, making it difficult to react in real time.
The problem with predefined reports
Even when data finally lands in a BI tool, there’s another problem: the reports are rigid.
Insurance professionals don’t always know exactly what they’re looking for. Today, an underwriter might need to compare premium collections vs. claims reserves, but tomorrow, they might want to look at policy adjustments vs. fraud indicators.
Traditional process and BI tools force them into predefined dashboards: static views that were built for yesterday’s questions, not today’s.
If they need a new perspective, they have to go through a painful request process:
- Find an analyst or IT team that manages reports.
- Explain what they need.
- Wait for that team to create a new view.
- Hope the new report actually answers their question.
By the time they get it, the moment to act has already passed.
Shifting analytics left: bringing insights closer to the data
This is where the real shift needs to happen. Instead of treating Kafka as just an event pipeline, organizations should use it as an analytical source: enabling decision makers to interact with real-time data directly, without waiting for batch jobs or static reports.
By moving analytics closer to Kafka, we unlock:
- True real-time insights: no more waiting for batch updates.
- Ad-hoc exploration: underwriters, reinsurers, and finance teams can ask questions as they arise, instead of waiting for IT to build a report.
- Dynamic decision-making: insurance professionals can react as the market changes, rather than making decisions on old data.
Think of it like having a live radar map instead of a weather report from yesterday. When storms are forming, when claims are rising, when risks are shifting, insurance professionals need the ability to see it happening in real time, not piece it together after the fact.
The future: underwriters, reinsurers, and finance teams in control
Insurance is an industry built on anticipating uncertainty. But for too long, insurers have created more uncertainty for themselves by relying on delayed, predefined analytics.
Underwriters don’t just need faster reports. They need the freedom to explore their data on their own terms.
Reinsurers don’t just need claims updates. They need continuous visibility into exposure and risk.
Finance teams don’t just need monthly cash flow projections. They need real-time tracking of payables, reserves and premium trends.
The future of insurance analytics isn’t just about speed, it’s about flexibility. It’s about enabling ad-hoc exploration, direct access to real-time data, and decisions that are made in the moment, not in hindsight.
It’s time to move analytics left, to bring decision-making as close to the data as possible. Because in this industry, uncertainty is already a given. The last thing we need is uncertainty in our data, too.